Reducing Downtime in Manufacturing with Predictive Analytics in today’s fast-paced manufacturing world, downtime is a dreaded enemy. Whether it’s scheduled or unscheduled, downtime can lead to significant losses in productivity, revenue, and customer satisfaction. But what if you could predict and prevent those unexpected disruptions? Enter predictive analytics a powerful tool that’s reshaping the manufacturing landscape.
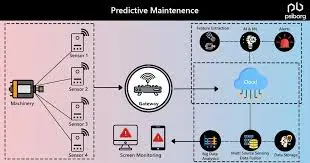
What is Downtime in Manufacturing?
Downtime refers to periods when production stops, whether due to machine failures, maintenance, or external factors. It’s broadly classified into two categories:
- Planned Downtime: Scheduled maintenance or upgrades.
- Unplanned Downtime: Sudden equipment failures or unexpected interruptions.
Unplanned downtime is particularly costly, often leading to ripple effects throughout the supply chain.
The High Cost of Downtime
Did you know that unplanned downtime costs industrial manufacturers an estimated $50 billion annually? For many companies, every minute of downtime means lost revenue and delayed deliveries, which can harm customer relationships.
Beyond financial implications, downtime also impacts employee morale, as workers may face pressure to compensate for lost time once operations resume.
Why Predictive Analytics Matters
Predictive analytics leverages data, algorithms, and machine learning to forecast future events. In manufacturing, it’s used to anticipate equipment failures, optimize maintenance schedules, and improve overall efficiency. By analyzing historical and real-time data, predictive analytics provides actionable insights that can:
- Reduce unplanned downtime.
- Extend the lifespan of machinery.
- Enhance operational efficiency.
- Lower maintenance costs.
How Predictive Analytics Works in Manufacturing
Predictive analytics involves several steps, each contributing to a seamless and proactive maintenance strategy:
1. Data Collection
Sensors embedded in machinery collect real-time data, such as temperature, vibration, and pressure. This data is then transmitted to a central system for analysis.
2. Data Processing
Once collected, the raw data is cleaned and organized. Advanced algorithms process this information, identifying patterns and anomalies.
3. Predictive Modeling
Using machine learning models, the system forecasts potential failures or inefficiencies. These models continuously improve as they’re exposed to more data.
4. Actionable Insights
Finally, the system provides actionable recommendations, such as scheduling maintenance or adjusting operational parameters.
Key Benefits of Predictive Analytics in Reducing Downtime
1. Proactive Maintenance
Traditional maintenance methods, like reactive and preventive maintenance, often fall short. Reactive maintenance fixes problems after they occur, while preventive maintenance relies on a fixed schedule, regardless of the equipment’s actual condition. Predictive analytics, on the other hand, enables maintenance based on real-time data, preventing issues before they arise.
2. Improved Equipment Efficiency
By identifying and addressing potential inefficiencies, predictive analytics ensures machinery operates at peak performance, minimizing wear and tear.
3. Enhanced Decision-Making
With predictive analytics, manufacturers can make data-driven decisions, from resource allocation to production scheduling, ensuring optimal outcomes.
4. Cost Savings
Reducing unplanned downtime and optimizing maintenance schedules translate into significant cost savings. Companies also save on spare parts and labor costs by addressing issues proactively.
Real-World Applications of Predictive Analytics
Predictive analytics isn’t just a futuristic concept; it’s already being implemented across industries:
Automotive Manufacturing
Major automotive manufacturers use predictive analytics to monitor assembly line equipment, reducing downtime and maintaining consistent production.
Food and Beverage Industry
Predictive analytics helps maintain stringent quality standards by ensuring equipment operates within specified parameters.
Aerospace Manufacturing
In this high-stakes industry, predictive analytics ensures critical machinery remains operational, reducing delays and enhancing safety.
Challenges in Implementing Predictive Analytics
While the benefits are undeniable, implementing predictive analytics comes with challenges:
1. Data Quality
Poor-quality data can lead to inaccurate predictions. Manufacturers must invest in reliable data collection systems.
2. Integration with Existing Systems
Integrating predictive analytics with legacy systems can be complex and time-consuming.
3. Skill Gaps
Many manufacturers lack the in-house expertise to implement and manage predictive analytics solutions.
Overcoming Implementation Barriers
To successfully adopt predictive analytics, manufacturers should:
- Invest in Training: Equip employees with the necessary skills.
- Partner with Experts: Collaborate with technology providers specializing in predictive analytics.
- Start Small: Begin with pilot projects to demonstrate value before scaling up.
Future Trends in Predictive Analytics for Manufacturing
As technology evolves, predictive analytics will become even more powerful. Some trends to watch include:
1. Integration with IoT
The Internet of Things (IoT) will enhance data collection, enabling more accurate predictions.
2. Artificial Intelligence (AI) Advancements
AI will further improve predictive models, making them faster and more precise.
3. Cloud-Based Solutions
Cloud computing will make predictive analytics more accessible, especially for small and medium-sized enterprises.
Case Studies: Success Stories
General Electric (GE)
GE’s use of predictive analytics in its manufacturing plants has reduced unplanned downtime by 20%, saving millions annually.
Siemens
Siemens employs predictive analytics to monitor equipment health, ensuring seamless operations and minimizing disruptions.
How to Get Started with Predictive Analytics
If you’re ready to reduce downtime with predictive analytics, here’s how to start:
- Assess Your Needs: Identify key areas where downtime impacts your operations.
- Choose the Right Tools: Select predictive analytics software tailored to your industry.
- Gather Data: Invest in sensors and data collection systems.
- Collaborate with Experts: Work with vendors or consultants to implement the solution.
Conclusion
Predictive analytics is revolutionizing manufacturing by transforming downtime from an unavoidable cost to a manageable variable. By investing in this technology, manufacturers can not only reduce downtime but also boost efficiency, cut costs, and stay competitive in an ever-evolving industry.
FAQs
1. What is predictive analytics in manufacturing?
Predictive analytics in manufacturing uses data and algorithms to forecast equipment failures, optimize maintenance, and enhance operational efficiency.
2. How does predictive analytics reduce downtime?
It identifies potential issues before they occur, allowing for proactive maintenance and minimizing disruptions.
3. Is predictive analytics expensive to implement?
While initial costs can be high, the long-term savings from reduced downtime and increased efficiency often outweigh the investment.
4. Can small businesses use predictive analytics?
Yes, cloud-based solutions and affordable tools make predictive analytics accessible for small and medium-sized manufacturers.
5. What industries benefit most from predictive analytics?
Industries like automotive, aerospace, food and beverage, and electronics have seen significant benefits from predictive analytics.